NVIDIA announced during the annual International Supercomputing Conference (ISC) the PCI Express version of the Tesla P100 accelerator.
Based on NVIDIA’s new Pascal architecture and their 16nm GP100 GPU, Tesla P100 is a step up from the Tesla K/M series and their respective 28nm Kepler/Maxwell GPUs. Besides being a bigger-still GPU, P100 introduces a number of new features including larger caches, instruction level preemptive context switching, and double speed FP16 compute.
"Accelerated computing is the only path forward to keep up with researchers' insatiable demand for HPC and AI supercomputing," said Ian Buck, vice president of accelerated computing at NVIDIA. "Deploying CPU-only systems to meet this demand would require large numbers of commodity compute nodes, leading to substantially increased costs without proportional performance gains. Dramatically scaling performance with fewer, more powerful Tesla P100-powered nodes puts more dollars into computing instead of vast infrastructure overhead."
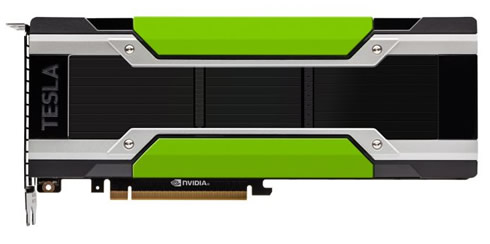
NVIDIA will be shipping two versions of the PCIe Tesla P100. The higher-end PCIe configuration has 56-of-60 SMs enabled, with a boost clock of 1.3GHz rather than the original P100’s 1.48GHz. This puts theoretical throughput at 9.3 TFLOPs for FP32 and 4.7 TFLOPs for FP64, versus 10.6 TFLOPs and 5.3 TFLOPs respectively for the original P100. In addition, whereas the mezzanine cards are 300W, the PCIe cards are 250W, which is the same TDP as past generation Tesla PCIe cards.
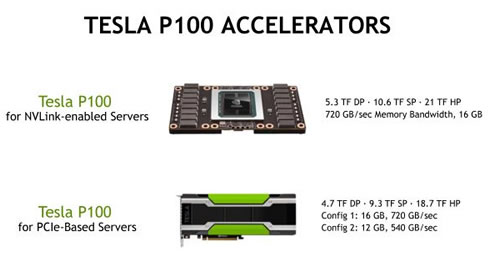
In addition, the higher-end card ships with the full 16GB of HBM2 enabled. It's 1.4Gbps HBM2 in a quad package configuration, allowing for 720GB/sec of bandwidth (both with and without ECC).
The lower-end card ships with the same GPU clockspeeds and overall compute throughput, but it cuts the amount of memory and the memory bandwidth by 25%. This brings the total memory capacity down to 12GB, and the total memory bandwidth down to 540GB/sec.
Both of these cards are going to be targeted at customers who either don’t need NVLink, or need drop-in card upgrades for current Tesla cards.
Nvidia said server makers like Cray, Dell and Hewlett-Packard Enterprise will start taking orders and delivering systems with the GPU starting in the fourth quarter this year.
NVIDIA has also reconfirmed that the Piz Daint supercomputer upgrade project is on schedule for later this year. The Swiss National Supercomputing Center will be doing a drop-in upgrade, replacing the supercomputer’s 4,500 Tesla K20X cards with Tesla P100 PCIe cards.
Software Updates
Along with the PCIe Tesla P100 announcement, NVIDIA is also announcing some software updates to components of their Deep Learning SDK, the company’s collection of various software libraries and tools.
NVIDIA DIGITS 4 introduces a new object detection workflow, enabling data scientists to train deep neural networks to find faces, pedestrians, traffic signs, vehicles and other objects in a sea of images. This workflow enables advanced deep learning solutions - such as tracking objects from satellite imagery, security and surveillance, driver assistance systems and medical diagnostic screening.
When training a deep neural network, researchers must repeatedly tune various parameters to get high accuracy out of a trained model. DIGITS 4 can automatically train neural networks across a range of tuning parameters, significantly reducing the time required to arrive at the most accurate solution.
DIGITS 4 release candidate will be available this week as a free download for members of the NVIDIA developer program. Learn more at the DIGITS website.
NVIDIA cuDNN provides high-performance building blocks for deep learning used by all leading deep learning frameworks. Version 5.1 delivers accelerated training of deep neural networks, like University of Oxford’s VGG and Microsoft’s ResNet, which won the 2016 ImageNet challenge.
Each new version of cuDNN has delivered performance improvements over the previous version, accelerating the latest advances in deep learning neural networks and machine learning algorithms.
cuDNN 5.1 release candidate is available today as a free download for members of the NVIDIA developer program.
The GPU Inference Engine is a high-performance deep learning inference solution for production environments. GIE optimizes trained deep neural networks for efficient runtime performance, delivering up to 16x better performance per watt on an NVIDIA Tesla M4 GPU vs. the CPU-only systems commonly used for inference today.
The amount of time and power it takes to complete inference tasks are two of the most important considerations for deployed deep learning applications. They determine both the quality of the user experience and the cost of deploying the application.
Using GIE, cloud service providers can more efficiently process images, video and other data in their hyperscale data center production environments with high throughput. Automotive manufacturers and embedded solutions providers can deploy powerful neural network models with high performance in their low-power platforms.
The NVIDIA Deep Learning platform is part of the broader NVIDIA SDK, which unites into a single program the most important technologies in computing today - artificial intelligence, virtual reality and parallel computing. |